‘Big data’ analytics – the collection and analysis of large amounts of data – is having a transformative impact on scientific research across disciplines. Although there is no single and consistent definition, there are three commonly agreed upon indicators of big data, the three ‘V’s: volume, velocity and variety. Volume refers to the massive amounts of data, velocity to the constant production and stream of data and variety to its unstructured nature. Machine learning (ML; initially teaching computers to learn and process information to ultimately perform tasks without out the need for further instruction) is a popular tool used, making connections and findings faster than humans.
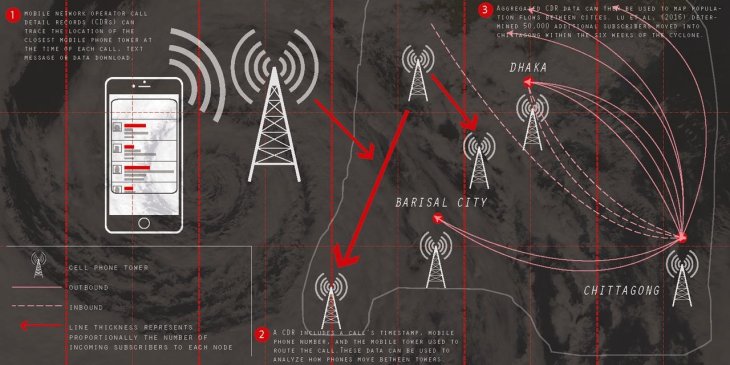
How cell-phone data can be used to map population movement. This example illustrates population flows after the 2013 Cyclone Mahasen in Bangladesh. Image courtesy of Shreya Shah (Yale University), published in PNAS.
A paper published by over twenty computer scientists (including those from DeepMind, Google AI and Harvard), highlights how machine learning could be utilized to tackle climate change, from climate modelling to climate impacts and adaptation.
For climate scientists, the utility of big data is not new. ML has seen use for some time in modeling the global climate system. But big data techniques are still emerging in the socioeconomic aspects of climate research, and here lies big potential for big data according to a recent paper published in Nature Climate Change. And by extension, I make the case below for how big data could have a positive impact on quantitative climate-conflict research. These implications should be tested in the future.
Using theory to structure big data analytics
In this Nature publication, the authors, Knüsel and colleagues, find that studies which structure their big data analysis through a theoretical lens, are able to contextualize their research and achieve greater impact. An example they point to is a study which aimed to estimate the impacts of Hurricane Sandy using geo-referenced Twitter data. By orienting this data using a socio-spatial theory, the authors were able to show how the twitter data became more relevant at certain spatial scales.
Buhaug has criticized the lack of theory informed quantitative analyses in the literature, saying “quantitative comparative literature is weak on theory, and explanations for observed patterns (or lack thereof) are often made post hoc”. ‘Pure’ big data by definition, lacks theoretical input and structure, which is to a degree an advantage. However, its unstructured nature seems to imply that Buhaug’s criticism risks being made worse. Examples like the above should be accounted for when attempting to integrate big data techniques into climate-conflict research.
Using proxies for missing data
Quantitative climate-conflict studies have moved towards using subnational as opposed to country level data. Among other benefits, this reduces the chances of missing important conflict related events and causal mechanisms at a local scale. However, such data can be difficult to collect and model.
An innovation with big data is that of developing proxies for missing data. One example sought to create an indicator that measured how vulnerable European cities are to climate change using Google search results as a proxy for the climate awareness of citizens.
Using this technique to measure events at local scales, as well as to measure complex population dynamics for which the data are difficult to collect, has potential. For example, as noted by Schleussner and colleagues, data at local scales that measure how natural disasters impact armed conflict risk is difficult to find. Financial transaction records (measuring population dynamics) and transport data (measuring the economic impacts of disasters) could act as a proxy for this hard to find data.
Additionally, if part of the ‘streetlight effect’ in climate-conflict research stems from a lack of accessible data in hard to reach areas as well as language and contact-based convenience in data collection (collection bias), then crowdsourced data through online questionnaires (acting as a conflict/climate events monitoring technique) could provide proxies to help fill this gap. See here for the usefulness in online questionnaires.
Big data and climate impact models
Buhaug notes that ‘complete’ theories in the climate-conflict literature are often too complicated to apply in empirical analyses, are incomplete and are not universally agreed upon. Therefore, constructing ‘theory-based models’ that inform how climate change impacts conflict risk is then considerably difficult to do. But, Knüsel and colleagues demonstrate that with enough background knowledge, new forms of big data could fill the gap in constructing and calibrating impact models, and machine learning is a useful tool to analyse these large and growing streams of data.
Making use of cell phone GPS data to help inform post climate impact migration patterns where such information holds value for those interested in understanding how these migration patterns impact on conflict risk. As noted by Salehyan in their special issue on climate and conflict, this could demonstrate how localized resource scarcity might cause conflict in other areas as affected populations migrate away towards resource rich areas thereby inducing resource stress on host populations. However, as noted earlier, this should not be seen as a replacement for theory, but it could be a way of bypassing areas where theory is ‘currently’ not transferable to empirical analyses.
Vulnerability assessments and conflict risk
Understanding how affected communities are vulnerable to climate change is crucial for forming appropriate adaptation and resilience building responses, and big data has serious potential to have a positive influence.
The importance of assessing conflict risk through vulnerability has been demonstrated here and here by Ide, Schilling and others, and these assessments are important for understanding ‘who’ is at an increased risk of conflict. In recent years the ‘who’ has moved from groups at the state level to smaller communal conflicts/groups, most often agricultural communities. It is important to understand these population dynamics, their sizes and how they react under climate stress as indicating conflict risk. For example, those communities who remain in their locale as opposed to migrating could indicate lower vulnerability and thus lower conflict risk. Financial or cell phone GPS data could prove useful in illuminating group mobility, as seen in this study.
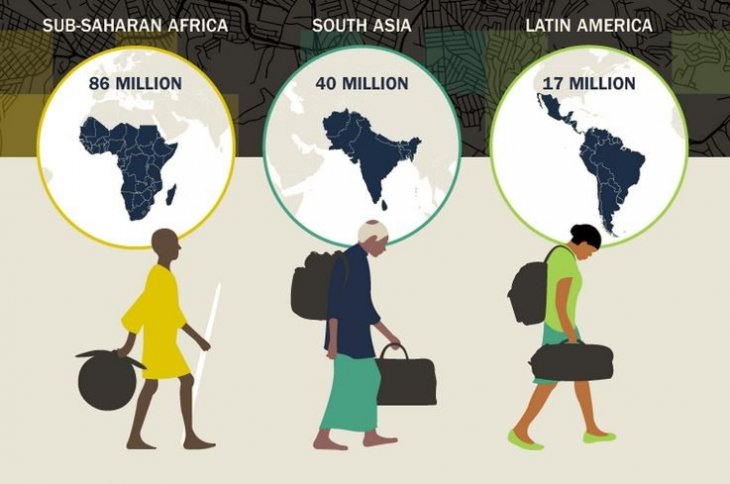
Possible numbers for internal migration in three climate-vulnerable regions. Big data techniques could help in understanding these population and migration dynamics. Source: The World Bank.
Developing climate services and reducing conflict risk
As noted above, increasing focus is being paid to agricultural groups in climate-conflict research. Building resilience and increasing their adaptive capacity is then not only important for their livelihood but also to reduce their susceptibility to conflict. The decision making for farming communities for example, depends on the utility of climate related information.
Machine learning can be used to combine differing types of data to help farmers better predict future climatic patterns, thereby highlighting better planting or crops, hence creating a useful climate service. This can function as an information service, helping farmers decide what adaptation methods to use and when, then increasing their climate and conflict resilience.
Moving forward
It is important not to think of big data and machine learning as an overarching fix to issues in the literature. And researchers should be wary of the potential to aggravate existing tensions where collecting sensitive data could be used by conflicting groups and authoritarian governments.
However, with thoughtful application and cooperation between climate-conflict researchers and data scientists, the above presents some promising avenues of research that could inform relevant decision making. Governmental and non-governmental organizations should seek to contribute to such efforts through research funding, potentially seeing positive dividends for society.
Daniel Pearson is a Graduate at Curtin University Sustainability Policy Institute (CUSP).
Leave a Reply