Kristin Bergtora Sandvik discusses the broader implications of evolving AI for humanitarian action, aid work, and aid workers.
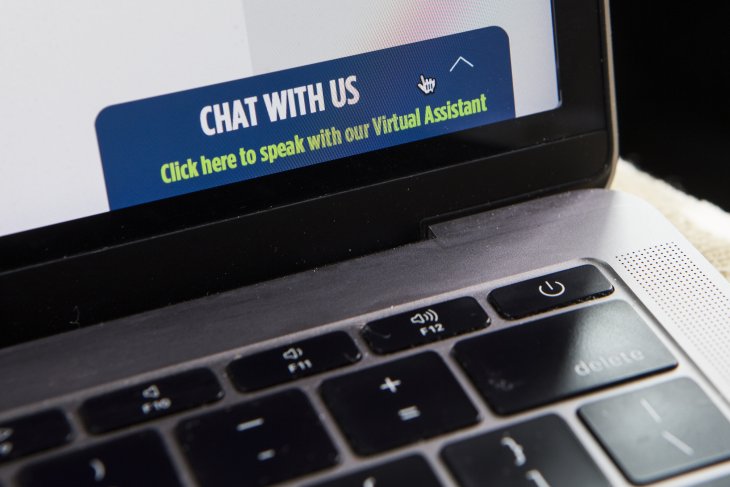
Photo: Brett Coomer/Houston Chronicle via Getty Images
Generative AI: From same, same but different to different
In the summer of 2023, the UN declared that the International Community Must Urgently Confront New Reality of Generative, Artificial Intelligence. The emergence of generative AI as a core issue in global governance also has institutional and logistical import for the humanitarian sector.
This is the second of a series of blog posts where we reflect on how the sector grapples with the challenges and opportunities of AI: The first blog discussed the framing of policy conversations around AI in humanitarian strategies. Taking the rise and rise of ChatGPT by OpenAI and Bard by Google as the point of departure, this blog takes stock of conversations around the broader implications for humanitarian work and workers. As before, the blog starts from a concern with discrimination, risk, and bias in the humanitarian sector and the need for a responsible and rights-based approach to AI.
Decades in the making, artificial intelligence is the ability of a computer system to imitate human thinking processes for problem-solving purposes. Using AI and machine learning algorithms, generative AI allows users to generate new content – words, images, videos – by giving prompts to the system to create specific outputs. Generative AI uses natural language processing and machine learning methods to parrot human communication. Highly experimental and arriving in an unprepared regulatory landscape, generative AI has within a short timeframe seen massive uptake globally. ChatGPT – short for Chatbot Generative Pre-Trained Transformer – by OpenAI was officially released in late 2022. Despite unresolved regulatory controversies, ChatGPT has become a household name. Several comparable products have been launched. The main competition is Bard by Google, using a different language model. Due to data protection regulation concerns, Bard was only rolled out in the EU in mid-summer 2023. For anyone interested, the search engine was advertised as: ‘New! Try Bard and an early AI experiment by Google.’ Similarly, image generators such as DALL-E (also by Open AI), Midjourney, and Stable Diffusion have engendered a flurry of concern around ethical and regulatory challenges.
After a decade of innovation talk and humanitarian technology hype cycles and about five to six years of incessant focus on the digital transformation of aid and AI, we are finally faced with what could be a real game changer. Together, these innovations, for the near future in perpetual beta testing phases, look poised to disrupt humanitarian programming, supply chains, and the everyday nature of aid distribution and protection. In the coming months, much will change with the technology itself as well as concerning how AI is adopted and adapted by the sector. Humanitarians must grapple with their assumptions about the technology, as well as the capacity of generative AI, its potential and actual applications in aid, and the potential and actual impact on the sector. As a contribution, through what is largely a sorting and framing exercise, this blog outlines three key conversations concerning the implications for humanitarian work. This includes the challenges of storytelling, information management, and representation; the politics of a toolbox approach to generative AI; and some evolving tensions between humanitarians working actively with AI and the prospect of AI as the humanitarian worker. The blog concludes that the adoption and adaptation of generative AI is a form of humanitarian experimentation and calls for revisiting discussions around humanitarian accountability.
Representing humanitarians: Storytelling, information management, and reputations
The first broad conversation is happening around representation and information. Historically, the quest to end human suffering is closely entangled with how aid actors are seen as succoring need. Public communication strategies and management of representation are central to humanitarian fundraising, programming, and aid delivery. Today, this includes the need for any organization to be able to present itself according to its values, policies, and mission. It also entails a need to understand what type of expected and inadvertent projections will arise from the public use of generative AI, including both by populations in crisis and those in donor countries.
Humanitarian communications and humanitarian photography remain fields in constant flux, where the need to inform and educate the public about the human impact of violence and suffering collides with ethics, changing cultural mores and reactions to past institutional colonialism, racism, and paternalism. AI adds further complexity to written and visual humanitarian storytelling and documentation. In a recent and rather spectacular example of failure, Amnesty International controversially used AI to generate images to protect Colombian protesters from possible state retribution. The intention was good, but the reception was bad.
In recent years, misinformation and disinformation have become key challenges for humanitarian field operations, and organizations have set up specific programs to monitor and mitigate misinformation flows, for example in the field of health. Information risk is likely to increase enormously in the future, ranging from the example of ChatGPT inventing a sex scandal and blaming a named individual, or falsely accusing named individuals of bribery, to market manipulation with the ability to sway the stock market and destabilize international trade, potentially even triggering conflict.
While evolving AI technology will mostly mean more of everything rather than something radically new, the impending relational impact of generative AI merits attention. With the multiple language versions offered by this technology, individual aid workers will now engage with local populations in the context of their digital personae as the various platforms collate, embellish, ignore, and mistake attributes. Previous postings, public engagements, and involvement in controversies will be visible to local communities in a very different way than what was previously the case. Moreover, at present, generative AI lies.
When preparing to write this blog post, the author asked Bard about her accomplishments. The response was glorious: she was awarded prizes and memberships in academic societies that she does not have. Then she asked ChatGPT for her profile. Despite numerous prompts, ChatGPT insisted that the author held a Ph.D. degree in social anthropology from the University of Oslo (false) and had no law degrees from either Norwegian or US universities (she does). For an academic, such mistakes may be of little consequence. Yet, had the author been a legal protection officer for UNHCR this would create a poor impression. If she had been a physician for MSF, a comparable denial of medical qualifications could create real issues of trust with the community.
Toolboxes and politics
The second conversation concerns the relationship between a toolbox approach to AI and the politics of AI. As humanitarian employers consider policies, hiring requirements, training needs, risk management, due diligence procedures, and liability issues, individual aid workers must also find their way with evolving AI tools. Better tools can do many things for humanitarians’ everyday work: An old colleague in the sector explained that ‘while ChatGPT can’t pick out good ideas from bad ones, it does help me to develop the ideas so that I can better evaluate them for potential use cases’. Similarly, humanitarian innovation processes could be made simpler, better, and more participatory. Prospective advantages range from providing streamlined procedures to facilitating the development, iteration, and evaluation of ideas and product developments (including about the needs of proposed users and how the innovation compares with existing products), undertaking market assessments, planning pilots, and so forth.
While offering opportunities for improvement, old challenges persist. For example, AI tools can provide a better understanding of patterns of violence, including faster and more accurate insights into who did what to whom and when, which will enhance protection work and the analysis of violent events that threaten the safety of humanitarian personnel. The promise of better data collection, management, and analysis is also the promise of analyzing and acting on data flows across humanitarian silos, ultimately achieving the breakdown of these silos. At the same time, any AI-generated actionable recommendation needs to be assessed for possible biases and blind spots due to gaps in data caused by digital divides; function creep of the data – or mission creep of the organization. Ultimately, however, these questions are political questions about power, values, and interest.
Along the same lines, administrative tasks such as budgeting, hiring, contracting, and analyzing tenders are continually digitized. Given the problems that may arise from design problems, human errors, and cyber-attacks, human oversight will be needed to oversee such operations. While problems of explicability, opacity, and transparency may make meaningful oversight challenging, channeling funding and decision-making power toward human-run humanitarian accountability mechanisms is more important than ever.
While many of these dilemmas, tradeoffs, and balancing acts belong to the mundane world of internal digital capacity building, there are some new challenges. As noted above, AI is not a magical truth machine. Users are encouraged to familiarize themselves with the technology by ‘asking about anything you are curious about’ to comprehend the potential and limitations of the tool, the workflow, and the impact on work and group dynamics. At present, the reply might regularly be that ‘I am just a language model’ and no answer can be provided. Yet, notably, as the technology also uses prompts and input for training, all types of questions including personal questions about oneself or colleagues, or specific, sensitive questions about host communities generate their own particular dynamics. If we take a cue from the advice tech employees get from their companies, such questions should not be asked.
An evolving dichotomy: Working with AI versus AI as a humanitarian worker
The third and final conversation deals with prospective tensions arising around working actively with AI as a tool versus the prospect of AI as a humanitarian worker.
The humanitarian sector presents a paradox regarding how we think about one of AI’s most negative perceived outcomes: job losses. According to the industry website, ‘Developmentaid.org’ rumors say that ChatGPT will replace many jobs. That is why now is the moment to secure your next job. Another actor suggests that: It is important for organizations and individuals using AI in Africa to carefully consider these risks and take steps to mitigate them.
Yet, on principle, the point of departure for thinking about the future of humanitarian work is somewhat different than for many other sectors: while some humanitarian professional groups are potentially made redundant or radically shrunk through AI, the prospect of rationalization looks principally different in a sector where the aspiration is for the humanitarian worker to be superfluous. The stated goal of any intervention is always that emergencies, wars, and crises will come to an end and humanitarians can exit (importantly, this question is different from that of cuts resulting from financial problems or the precarity of local humanitarian labor).
Another issue concerns grant writing and the potentially equalizing impact of generative AI. Several commentators see the potential of AI to take care of the dull, if not the dangerous, and dirty aspects of humanitarian work. In particular, this concerns grant writing and project proposals. For example, it is suggested that ChatGPT could really open doors for smaller organizations that can’t afford proposal writers. To respond to this shift – which will likely result in a deluge of professional-looking and very similar applications, bids, and tenders – donors must understand what they need to know, changing their evaluation criteria to focus more on technical knowledge, experience, and an understanding of the context that can’t be sourced from the internet.
But will they? Awards to fraudulent actors or bad project is one thing, but is there also a risk that the importance of informal networks, personal ties, and established prestige and credibility becomes even more important for accessing funding than it already is, resulting in de-localization of procurement and entrenching current disparities in the aid sector?
A further issue concerns intelligibility and shared understandings in a global context seeing increasing authoritarianism and digital censorship. How will generative AI impact the engagement between humanitarians and communities?
On one hand, the long-lamented trends of bunkerization and remote management will likely continue. At the same time, language technology is already changing how aid workers communicate with the populations they serve. While this opens up significant possibilities for enhancing effectiveness and efficiency, humanitarians must also be able to know what they are doing and communicating – and how built-in surveillance, fencing, and manipulation feature in this process. What this means for language and cultural competence as a skillset favoring local employees is unclear. Moreover, because the adoption and adaptation of generative AI will be a two-way street in the humanitarian space, the difference between the free and the paid-for tools is significant. Humanitarians should reflect on what this quality discrepancy might entail for marginalized groups using free software.
Concluding thoughts
This blog has mapped out three broad conversations around generative AI happening in the aid sector. One of the interesting aspects of these conversations is the broad recognition that it is here. In terms of the humanitarian workforce, generative AI entails an expansion of the digital literacy requirement in the sector, while also promulgating a sense of fear and urgency. Old dilemmas persist – data and cybersecurity issues are not going away –and some new ones are on the horizon.
As illustrated by this post, the change engendered by generative AI appears slated to be different than what is usually promised by either salvational or dystopian tech talk: the change is both mundane and incremental – and represents a fundamental yet little-understood disruption of humanitarianism. In the context of the digital transformation of aid, imaginaries of failure regularly amount to utopianist and fantastical. Yet, as has been noted, while AI systems can exceed human performance in many ways, they can also fail in ways that a human never would.
While this technology is still in its infancy, at its core, the adoption and adaption of generative AI already amount to a comprehensive and unprecedented mainstreaming of humanitarian experimentation across the aid sector. Humanitarian organizations and their employees must recognize this and strike a balance between proactive adoption and responsible training and use while analyzing what generative AI means for their missions and their everyday work. This will also require new understandings of and approaches to humanitarian accountability.
- Kristin Bergtora Sandvik (S.J.D Harvard Law School 2008) is a professor of legal sociology at the Faculty of Law, University of Oslo, and a Research Professor in Humanitarian Studies at PRIO. Sandvik’s research on the humanitarian sector focuses on refugee resettlement, legal mobilization, technology, innovation, and accountability. Her new book, Humanitarian Extractivism: The digital transformation of aid is forthcoming with Manchester University Press.
- This blog is an output of Do No Harm: Ethical Humanitarian Innovation and Digital Bodies, funded by the Research Council of Norway. I am grateful to Kristoffer Lidén for valuable comments and to Linnet Taylor for inspiration.