Driven by the ever-increasing availability of (big) data, as well as computational power and resources, we are currently witnessing an important academic debate about the promises and pitfalls of predicting social and human behavior.
Given its potentially disastrous consequences, the prediction of armed conflict and political violence more generally, not surprisingly takes a central place in this discussion.
Why should we welcome this debate, what is the current state of the art, and what are some key future challenges?
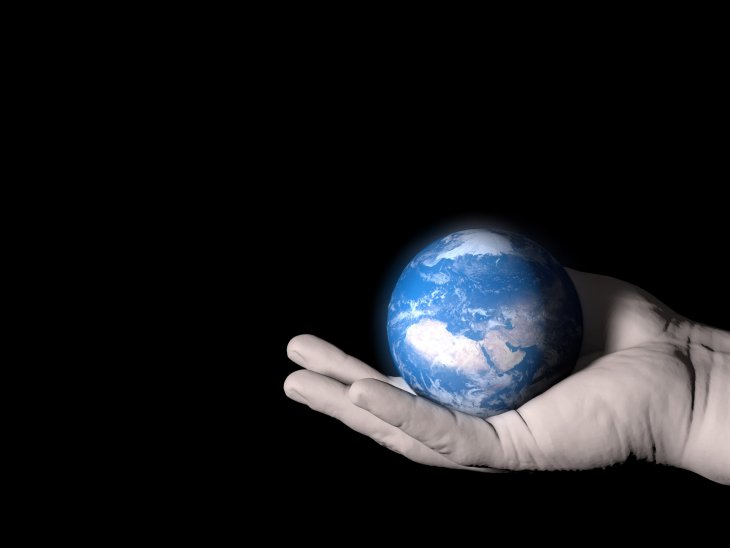
CC0 Public Domain. PublicDomainPictures.net
A special issue on forecasting in peace research published earlier this year in the Journal of Peace Research addresses these and other issues. The guest editors here offer a brief summary, as well as some additional reflections.
In 1973, J. David Singer, one of the pioneers of the field of peace research, argued that “the number one task of peace research always turns out to be that of prediction”. Singer believed that scientifically rigorous knowledge about the causes, dynamics, and consequences of peace and conflict would enable researchers to “forecast, with increasing reliability, the outcomes which are most likely to emerge out of a given set of background conditions”. This would ultimately help communities and policy makers to prevent, or at least mitigate, the scourge of armed conflict. Although Singer’s intentions were genuine and honorable, the task proved overly ambitious. Peace science at the time lacked both the theories and the data to forecast armed conflict with a useful degree of accuracy. Consequently, the ambition to forecast was mostly dropped altogether. In this, peace science to a large extent also sacrificed its determination to be directly policy relevant.
Lately, this has changed. Starting in the 1970s, but then accelerated by the ground-breaking work of the State Failure Task Force, later re-named the Political Instability Task Force, forecasting has become an increasingly active sub-field. This trend went hand in hand with the realization that the standard mode of scientific inquiry, null-hypotheses significance testing, faces limitations when it comes to producing policy relevant knowledge, since statistical significance is not an attribute of prediction. As an alternative or complementary route, a clear focus on prediction is increasingly used throughout the discipline of peace and conflict research.
The special issue on forecasting in peace research, published in the Journal of Peace Research earlier this year is testimony to this development. The contributions demonstrate that scientific forecasting of conflicts has made enormous progress in the past years, while providing a comprehensive impression of how political scientists approach this topic. Much progress in conflict forecasting stems from new methods and data, which enables almost real-time forecasts that are policy relevant.
We forecast that forecasting will continue to grow in the coming years. The most important questions regarding the future of forecasting in peace research pertain to its shape, not its importance. We believe that the interplay between theory and forecasting will increasingly take place along data and methods development as crucial elements of forecasting approaches in our discipline. While data-driven approaches will play a greater role in early-warning models, theory-driven models will remain crucial to determine factors that can be subject to policy intervention, thereby offering more specific policy guidance.
Table of contents
- Forecasting in Peace Research (Håvard Hegre, Nils W Metternich, Håvard Mokleiv Nygård, Julian Wucherpfennig)
- Using night light emissions for the prediction of local wealth (Nils B Weidmann, Sebastian Schutte)
- Lessons from near real-time forecasting of irregular leadership changes (Michael D Ward, Andreas Beger)
- Canaries in a coal-mine? What the killings of journalists tell us about future repression (Anita R Gohdes, Sabine C Carey)
- Subnational violent conflict forecasts for sub-Saharan Africa, 2015–65, using climate-sensitive models (Frank DW Witmer, Andrew M Linke, John O’Loughlin, Andrew Gettelman, Arlene Laing)
- Do the robot. Lessons from machine learning to improve conflict forecasting (Michael Colaresi, Zuhaib Mahmood)
- Financing rebellion. Using piracy to explain and predict conflict intensity in Africa and Southeast Asia (Ursula Daxecker, Brandon C Prins)
- The oracle or the crowd? Experts versus the stock market in forecasting ceasefire success in the Levant (Gerald Schneider, Maya Hadar, Naomi Bosler)
- Evaluating the scope and intensity of the conflict trap. A dynamic simulation approach (Håvard Hegre, Håvard Mokleiv Nygård, Ranveig Flaten Ræder)
- Predicting the decline of ethnic civil war. Was Gurr right and for the right reasons? (Lars-Erik Cederman, Kristian Skrede Gleditsch, Julian Wucherpfennig)
- The shape of things to come? Expanding the inequality and grievance model for civil war forecasts with event data (Daina Chiba, Kristian Skrede Gleditsch)
- Predicting local violence. Evidence from a panel survey in Liberia (Robert A Blair, Christopher Blattman, Alexandra Hartman)
- Market anticipations of conflict onsets (Thomas Chadefaux)